Tokenization in NLP: Breaking Language into Meaningful Words
Naveen
- 0
Tokenization is a fundamental concept in Natural Language Processing (NLP) that involves breaking down text into smaller tokens. Whether you’ve heard of tokenization before or not, this article will help you get the clear and concise explanation.
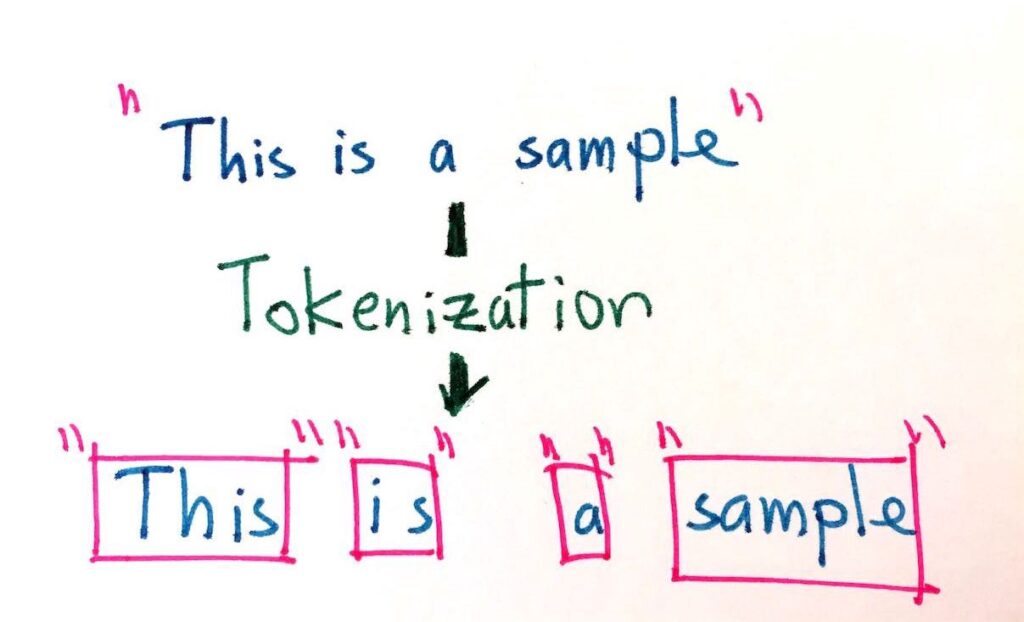
What is Tokenization?
Tokenization is the process of dividing a given text, such as a document, paragraph, or sentence, into individual words or units called tokens. We use these tokens as the building blocks for further analysis and processing in NLP tasks.
Understanding Tokenization
Let’s look at a simple example to explain the concept of tokenization. Imagine you have a document that contains several paragraphs, sentences and words. For simplicity, we will focus on one paragraph. The purpose of a token is to break this part into its constituent parts, where each word represents a token. This process of dividing text into characters is called tokenization.
Tokenization in Practice
There are various tools and libraries available for tokenization in NLP. One commonly used tool is the nltk.word_tokenize() function, which effectively splits a sentence into individual words or characters. Using this feature, we can easily split sentences into component tags for further analysis and processing.
Also, if we need to segment the text into sentences and determine the length of each sentence, we can use the nltk.sent_tokenize() function to tokenize the sentences and use the len() function to calculate the length of each sentence.
Let’s look at the example:

Conclusion
Tokenization plays a important role in NLP by breaking down text into meaningful units or tokens. These tokens are essential for various NLP tasks, such as text classification, sentiment analysis, and machine translation. By using tools like nltk.word_tokenize() and nltk.sent_tokenize(), we can effectively perform tokenization at both the word and sentence levels.
Popular Posts
Author
-
Naveen Pandey has more than 2 years of experience in data science and machine learning. He is an experienced Machine Learning Engineer with a strong background in data analysis, natural language processing, and machine learning. Holding a Bachelor of Science in Information Technology from Sikkim Manipal University, he excels in leveraging cutting-edge technologies such as Large Language Models (LLMs), TensorFlow, PyTorch, and Hugging Face to develop innovative solutions.
View all posts